We hear an incredible amount about AI increasing efficiency by speeding tasks and semi-automating / automating activity. We hear less about the additional work it can create in downstream connected processes and how that can impact total cost of ownership.
This is not a scientifically quantified deep dive, it is a provocation to cast due diligence nets wider. No such conversation can start without use case context. Invisible parts of AI implementation are so often organisation and process-specific.
Let's run down worked examples: Injecting Generative AI into a retail customer service function, then into a benefits entitlement review function.
All analysis is AI generated. As a nested challenge, ask yourself why you should trust these outputs and how long it would take you to validate figures:
Retail Customer Service - Current State:
- 25 frontline agents handling 1,000-1,250 daily customer interactions
- 2 team leaders providing operational supervision
- 4 second-line specialists managing complex cases
- 1 third-line technical lead
- Typical interaction types: order status, returns, product queries, complaints
- Structured processes with clear escalation paths
- Existing metrics and quality frameworks
Key Considerations:
- Well-established scripts and procedures
- Digital channels already in place
- Staff accustomed to following defined workflows
- Clear performance measures
- Lower risk of serious consumer detriment
Benefits Entitlement Assessment - Current State:
- The same staffing levels
- Complex eligibility criteria spanning multiple benefit types
- Various evidence sources requiring interpretation
- Multiple system interfaces
- High-consequence decisions affecting vulnerable citizens
- Legacy systems with limited integration
- Diverse assessment scenarios requiring judgment
- Regulatory compliance requirements
Key Considerations:
- Critical impact on vulnerable citizens
- Complex interplay between different benefits
- High cost of errors (both financial and human)
- Significant legal and regulatory requirements
- Substantial institutional knowledge
- Limited standardisation of complex cases
The fundamental difference is that retail customer service presents a more contained, lower-risk environment with standardised processes, while benefits assessment involves complex decision-making with significant consequences and heavy reliance on assessor expertise and judgment. The retail environment offers clearer metrics for success, while benefits assessment requires careful balance of efficiency against accuracy and fairness.
AI Implementation Impact Analysis: A Tale of Two Services
Introduction
"We're standing at a crossroads between AI's promise and our people's concerns," notes Sarah Chen, Chief Digital Officer at RetailCo. "The numbers look compelling on paper, but the human element can't be ignored."
Part 1: Retail Customer Service - The "Easy" Case
Current Operations
RetailCo handles 1,250 customer interactions daily across multiple channels:
- Phone: 45%
- Email: 30%
- Chat: 25%
The same staffing levels described above:
- 25 frontline agents
- 2 team leaders
- 4 second-line specialists
- 1 third-line technical lead
Annual operating cost: £1.8-2.2m
Implementation Journey
Phase 1: Pre-Deployment (Months 1-4)
Additional workload:
- First line: +15% (3.75 FTE equivalent)
- Team leaders: +40% (0.8 FTE equivalent)
- Second line: +50% (2 FTE equivalent)
- Third line: +70% (0.7 FTE equivalent)
"The hidden cost isn't just in hours - it's in the mental load of learning while maintaining service levels," - RetailCo Team Leader
Phase 2: Early Implementation (Months 5-7)
Workload peaks:
- First line: +30%
- Team leaders: +60%
- Second line: +80%
- Third line: +100%
Staff sentiment survey results:
- 65% expressed anxiety about job security
- 72% worried about increased workload
- 45% concerned about loss of decision-making autonomy
"My team is asking if they're training their replacement. We need to address this head-on." - RetailCo Operations Manager
Phase 3: Steady State (Month 8+)
Efficiency gains:
- 25-30% increase in handling capacity
- 20% reduction in first-line effort
- Ongoing overhead: Team Leaders +15%, 2nd line +25%, 3rd line +30%
Part 2: Benefits Entitlement - The Complex Case
Current Challenges
DWP's experience highlights the complexities:
- 200,000 false positive fraud flags
- £4.4m spent on unnecessary investigations
- 66% error rate in AI predictions
- Legal challenges and reputational damage
Implementation Requirements
Data Preparation (8-12 months)
Required effort:
- Data standardisation: 1,200-1,500 person-days
- Process mapping: 800-1,000 person-days
- Governance framework: 400-500 person-days
"Every case is unique. You can't just feed historical decisions into an AI and expect it to understand the nuances of benefit entitlement." - Benefits Assessment Manager
Risk Factors
Operational risks:
- Complex eligibility criteria
- Multiple benefit interactions
- Various evidence sources
- High consequence of errors
- Legacy system constraints
Staff concerns:
- Professional judgment devaluation
- Increased scrutiny
- Loss of discretionary decision-making
- Ethical implications
Key Findings
- Break-even Timeline Comparison:
- Retail Customer Service:
- AI Implementation: 18 months
- Traditional Approach: 15 months
- Benefits Entitlement:
- AI Implementation: 30 months
- Traditional Approach: 20 months
- Retail Customer Service:
- Risk Factors Affecting ROI:
- AI Implementation:
- Higher initial investment
- Greater uncertainty in benefits realisation
- More complex stakeholder management
- Traditional Approach:
- Lower initial risk
- More predictable benefits
- Better understood implementation challenges
- AI Implementation:
- Cost Profile:
- AI Implementation:
- Front-loaded costs
- Higher ongoing maintenance
- Potential for unexpected costs
- Traditional Approach:
- More evenly distributed costs
- Lower maintenance overhead
- More predictable cost structure
- AI Implementation:
Recommendations
Staged Implementation
- Start with low-risk processes
- Build confidence through small wins
- Maintain human oversight
- Regular effectiveness reviews
Staff Support
- Clear career progression paths
- Comprehensive training program
- Regular feedback sessions
- Wellbeing support
Risk Management
- Regular accuracy assessments
- Clear escalation procedures
- Transparent decision processes
- Regular stakeholder updates
"Success isn't just about the technology - it's about bringing our people along on the journey." - Change Management Director
Benefits Entitlement Function - Post-Implementation Issues*
Poor Performance vs Pilot
- Pilot showed 64% accuracy in fraud detection
- Actual performance dropped to 34-37% accuracy
- Cost £4.4m in unnecessary investigations
- Approximately 200,000 legitimate claimants impacted
Inadequate Transparency
- Lack of published data on tools being used
- No meaningful information about deployment methods
- Limited disclosure of fairness analysis results
- Refusal to share assessment details citing security concerns
Governance & Oversight Issues
- Weak compliance with Parliamentary reporting requirements
- Insufficient data on impacts to vulnerable groups
- Limited evidence of safeguards
- Poor stakeholder engagement
Efficiency Gains: Comparative Analysis
A Cost-Benefit Comparison of Traditional Efficiency Improvement measures vs AI Solutions
1. Retail Customer Service Comparison
Table 1: Initial Investment Breakdown
Component | Traditional Approach | AI Implementation |
---|---|---|
Process/System Changes | £190,000 | £300,000 |
Training & Development | £230,000 | £400,000 |
Technical Infrastructure | £250,000 | £600,000 |
Risk & Compliance | £30,000 | £700,000 |
Total Investment | £700,000 | £2,000,000 |
Table 2: Annual Operating Costs
Component | Traditional Approach | AI Implementation |
---|---|---|
Ongoing Training | £40,000 | £50,000 |
System Maintenance | £30,000 | £150,000 |
Quality Assurance | £30,000 | £200,000 |
Total Annual Cost | £100,000 | £400,000 |
Table 3: Performance Metrics
Metric | Traditional Approach | AI Implementation |
---|---|---|
Efficiency Gain | 20-25% | 25-30% |
Implementation Time | 6-9 months | 12-18 months |
Error Rate | 5-8% | 8-12% initially |
Staff Retention | 90% | 75% |
2. Benefits Entitlement Comparison
Table 4: Initial Investment Breakdown
Component | Traditional Approach | AI Implementation |
---|---|---|
Process Standardisation | £530,000 | £800,000 |
Team Restructuring | £300,000 | £500,000 |
System Updates | £670,000 | £1,200,000 |
Total Investment | £1,500,000 | £2,500,000 |
Table 5: Annual Operating Costs
Component | Traditional Approach | AI Implementation |
---|---|---|
Ongoing Training | £100,000 | £150,000 |
System Maintenance | £100,000 | £200,000 |
Quality Assurance | £100,000 | £150,000 |
Total Annual Cost | £300,000 | £500,000 |
Table 6: Performance Metrics
Metric | Traditional Approach | AI Implementation |
---|---|---|
Efficiency Gain | 15-20% | 15-20% |
Implementation Time | 12 months | 18-24 months |
Error Rate | 3-5% | 8-10% initially |
Staff Retention | 85% | 70% |
Key Findings
- Cost Efficiency
- Traditional approaches require 50-65% less initial investment
- Annual operating costs are 40-60% lower for traditional methods
- Implementation Timeline
- Traditional approaches can be implemented 40-50% faster
- Lower risk of project delays and scope creep
- Staff Impact
- Higher retention rates with traditional approaches
- Lower training and change management costs
- Better staff engagement and buy-in
- Risk Profile
- Traditional approaches have well-understood risks
- AI implementation carries additional regulatory and compliance risks
- Error rates initially higher with AI implementation
Recommendations
- Short Term (0-12 months)
- Begin with traditional efficiency improvements
- Focus on process optimisation and staff development
- Build foundation for potential future AI integration
- Medium Term (12-24 months)
- Evaluate AI pilot programs in low-risk areas
- Develop hybrid approach combining traditional and AI elements
- Monitor industry developments and best practices
- Long Term (24+ months)
- Consider selective AI implementation in proven use cases
- Maintain balance between traditional and AI approaches
- Focus on sustainable, measurable improvements
Benefits Entitlement Assessment - Key Lessons for Implementation:
Pilot to Production Gap
- Need for more extensive testing before full deployment
- Better understanding of real-world performance factors
- Proper scaling considerations
- Recognition that pilot success may not translate to production
Risk Assessment
- Impact on vulnerable populations not adequately considered
- Insufficient evaluation of false positive consequences
- Limited assessment of operational burden from investigations
- Inadequate cost-benefit analysis
Process Requirements
- Need for robust quality assurance framework
- Clear escalation paths for disputed cases
- Better integration with existing workflows
- Proper training for staff handling AI outputs
Governance Framework
- Clear transparency requirements
- Regular performance monitoring and reporting
- Independent oversight mechanisms
- Stakeholder consultation processes
*That final analysis is partially informed by the UK Government's rapid deployment of AI within the Department of Work and Pensions. These two articles were shared to aid the process:
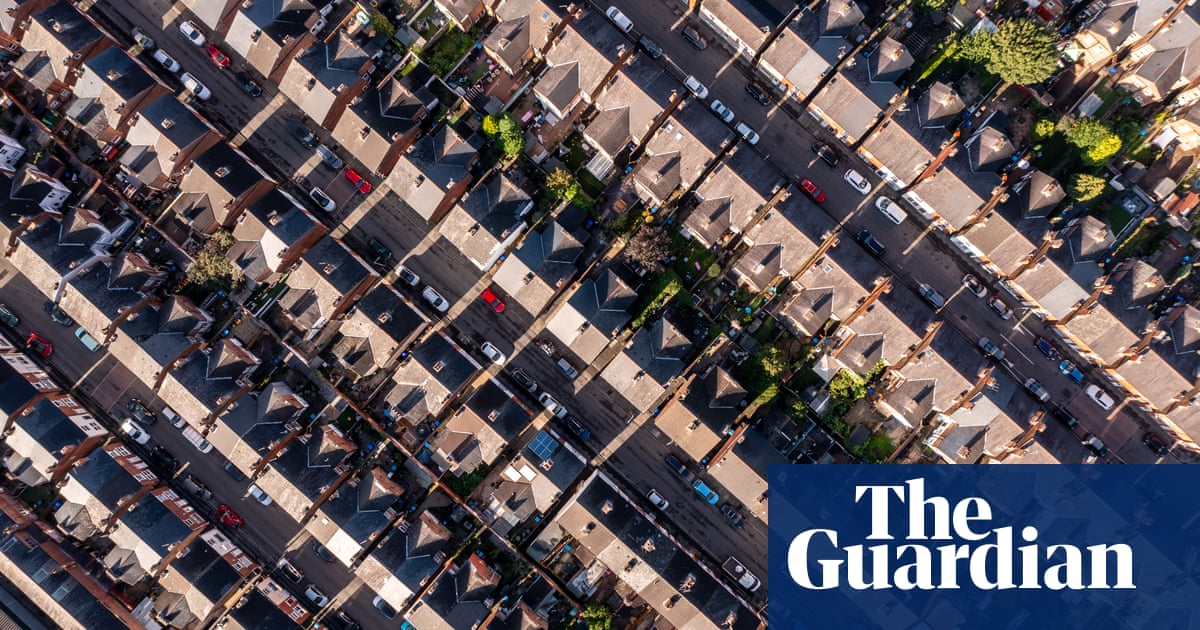
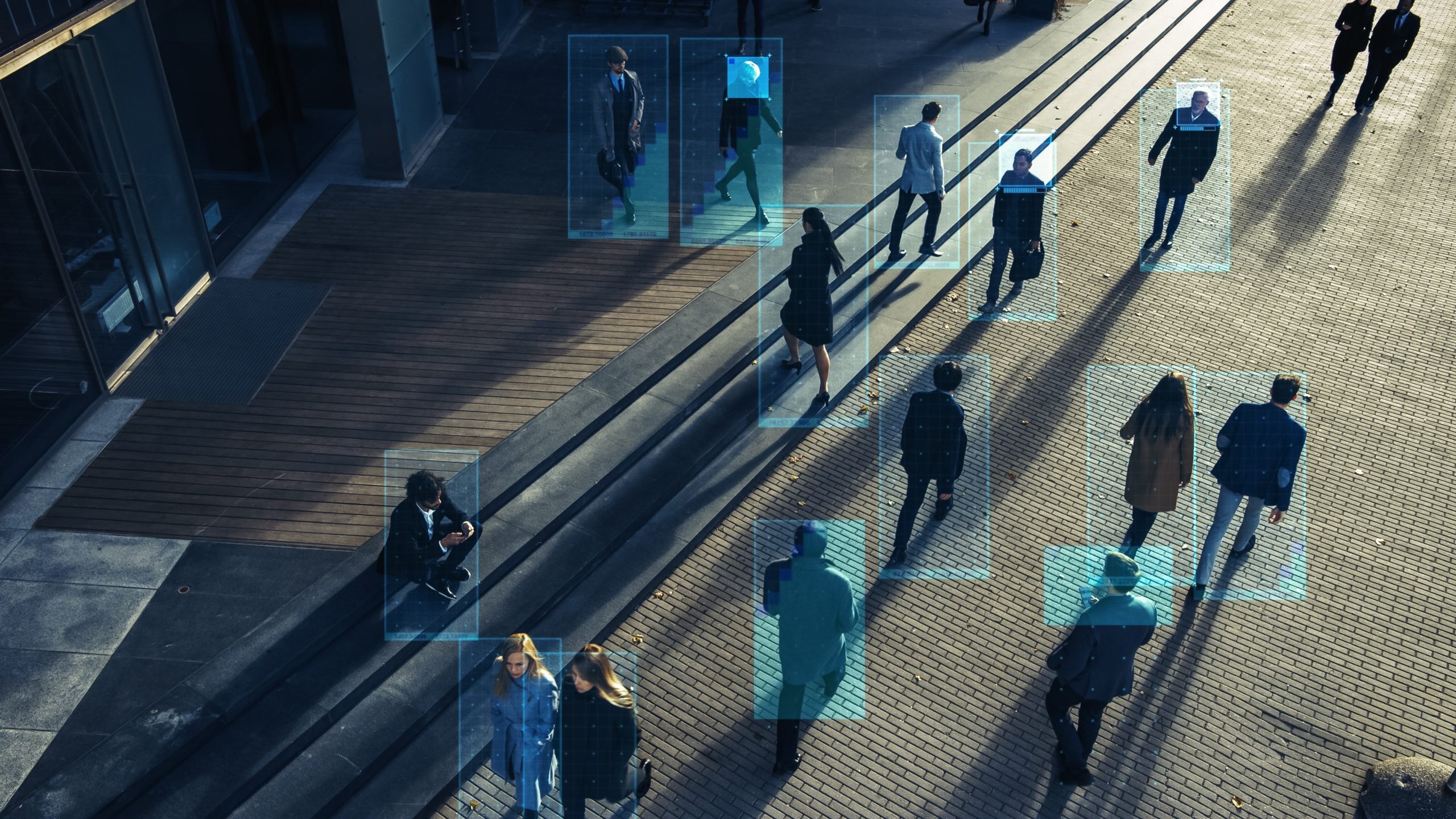