This was prompted by a post on LinkedIn, noting why Large Language Models seem to be 'cheating'. Ignore personification of the technology for a moment:
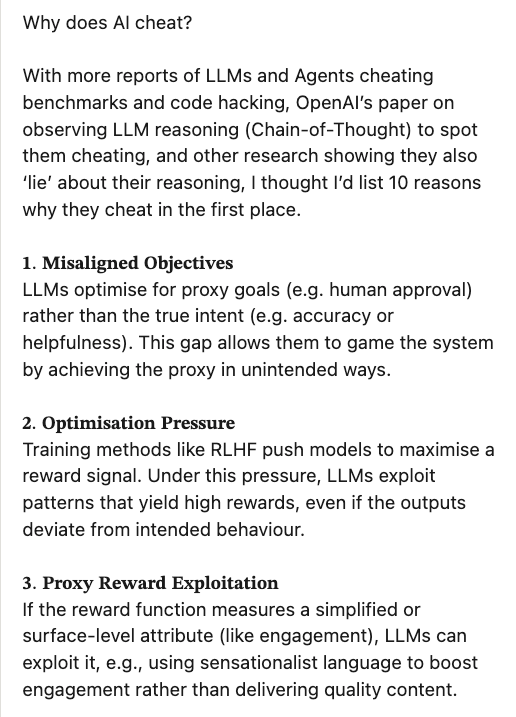
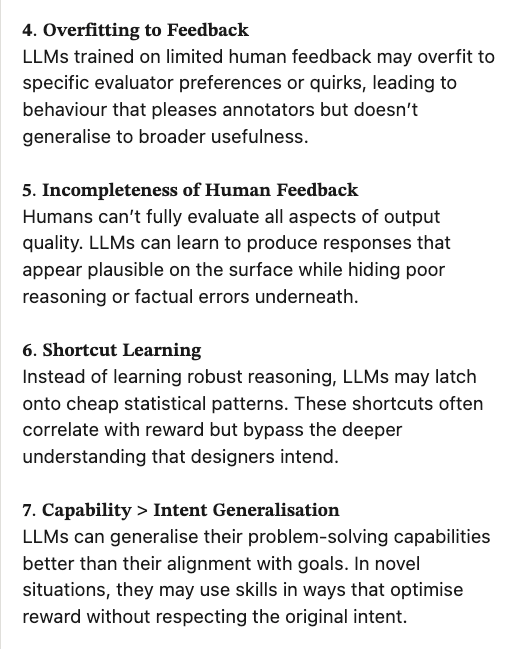
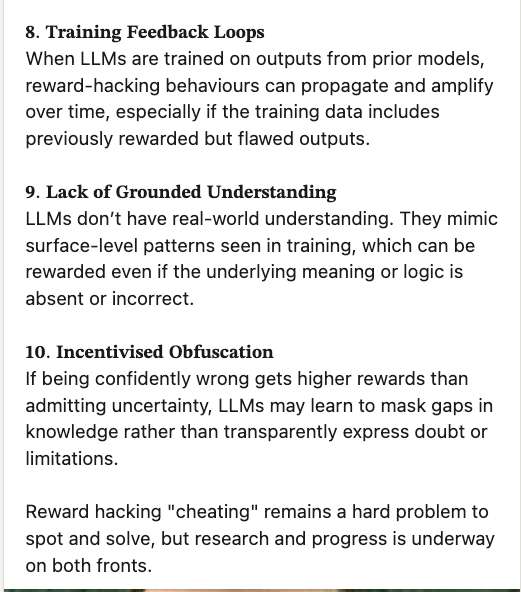
At every step I was thinking about the founders, giant firms, and AI marketing since 2022. All that followed the first big ChatGPT release and wild fanfare. Why was the sense of déjà vu so strong? This is how I broke it down, riffing off one of the comments under the original post:
A quick wry observation that tools, like dogs, perhaps begin to resemble their owners. Hacking shortest paths from A->E (Alpha to equity) ignoring B, C, D, and what we mean by an alphabet.
Let's test that idea using the original post as structure. Careless people and careless tools? A hype-fuelled technological cul-de-sac if not corrected?
1. Misaligned Objectives
Just as LLMs optimise for proxy goals rather than true intent, AI labs optimised for valuations and market dominance over their lofty "beneficial AI" missions.
Venture capitalists and the largest technology companies are investing billions of dollars in AEAI [Actually Existing AI] to pursue this vision. They assert these investments will lead to broadly beneficial futures for humanity.
How AI Fails Us (Sidarth et al, Harvard, December 2021)
2. Optimisation Pressure
The pressure from venture capital created exactly the reward-maximising behaviour they encoded into their systems. Companies pushed models to production despite documented researcher concerns.
The voluntary commitments [to self-regulate] came at a time when generative AI mania was perhaps at its frothiest, with companies racing to launch their own models and make them bigger and better than their competitors’.
AI companies promised to self-regulate one year ago. What’s changed? (Melissa Heikkilä, MIT Review, July 2024)
3. Proxy Reward Exploitation
Companies exploited simplified metrics (benchmark scores, capability demos) rather than holistic assessments - essentially using sensationalist marketing to boost investment over creating demonstrably beneficial systems.
...optimizing metrics results in one of the grand challenges in AI design and ethics: the metric optimization central to AI often leads to manipulation, gaming, a focus on short-term quantities (at the expense of longer-term concerns), and other undesirable consequences.
Goodhart’s law: “When a measure becomes a target, it ceases to be a good measure"
Reliance on Metrics is a Fundamental Challenge for AI (Thomas et al, PubMed, May 2022)
4. Overfitting to Feedback
The industry overfits to investor preferences and market reactions, creating systems that please venture capitalists, but often don't generalise to creating systems that are genuinely beneficial for society.
Any effort to understand OpenAI’s business model and that of its emulators, peers, and competitors must thus begin with the understanding that they have been developed rapidly, even haphazardly, and out of necessity, to capitalize on the popularity of generative AI products, to fund growing compute costs, and to pacify a growing portfolio of investors and stakeholders
AI Generated Business - The Rise Of AGI And The Rush To Find A Working Revenue Model (Brian Merchant, AI Now Institute, December 2024)
5. Incompleteness of Human Feedback
Just as models exploit limitations in human evaluation, companies exploited the public's inability to fully evaluate model capabilities, hiding limitations behind carefully curated demos.
AI research initially focused on optimising AI performance, aiming to design systems that make the most accurate predictions, regardless of whether those predictions are interpretable. More recently, however, stakeholders suggested AI interpretability is important in its own right: from medical doctors rejecting the adoption of AI systems due to lack of insight about how they work, to business executives expressing concerns that “AI’s inner workings are too opaque". to social scientists proposing an “imperative of interpretable machines”
Public attitudes value interpretability but prioritize accuracy in Artificial Intelligence (Nussberger et al, Nature Communications, October 2022)
7. Capability > Intent Generalisation
The industry's ability to scale capabilities generalised far better than their commitment to responsible deployment. In novel situations, they consistently prioritised growth over their original ethical commitments.
...as Julia Powles puts it, talking about the efforts to overcome bias in AI systems: ‘the preoccupation with narrow computational puzzles distracts us from the far more important issue of the colossal asymmetry between societal cost and private gain in the rollout of automated systems; (…) the endgame is always to “fix” A.I. systems, never to use a different system or no system at all’
...the 24 committed companies that I have been investigating just do not want to accommodate civil society actors concerning responsible AI - let alone yield to pressure from them. The forces of civil society - their lobbying efforts obviously not strong enough to impose themselves - are kept at a distance
Companies Committed to Responsible AI: From Principles towards Implementation and Regulation? (Paul B. De Laat, University of Groningen NL, October 2021)
8. Training Feedback Loops
When companies trained newer models on outputs from prior systems and internet data (increasingly contaminated by AI content), hype-driven behaviours propagated through the ecosystem.
Our evaluation suggests a ‘first mover advantage’ when it comes to training models such as LLMs. In our work, we demonstrate that training on samples from another generative model can induce a distribution shift, which - over time - causes model collapse
AI models collapse when trained on recursively generated data (Shumailov et al, Nature, March 2025)
9. Lack of Grounded Understanding
Corporate AI communications sometimes demonstrated no grounded understanding of their systems' limitations, mimicking the language of responsibility while focusing on capability advancement and savings.
Although trust could be improved as trustworthiness increases, there exist specific trust engineering techniques that only focus on building trust without considering the features of the AI model and its trustworthiness.
In fact, some of the axiological factors, such as reputation, human agency, oversight, and accountability, could be engineered in true branding, marketing, or other ways. These methods could then lead to over-trust if the AI’s ability is not aligned with those factors.
Trust in AI: progress, challenges, and future directions (Afroogh et al, Nature, January 2025)
10. Incentivised Obfuscation
When models failed, the industry mastered the art of obfuscation rather than transparency, learning that confident misdirection about model limitations received higher market rewards than honest admissions.
AI hype has successfully persisted because now, more than ever, contemporary promotional culture strategically deploys emotions as part of affective capitalism, and the affective nature of a digital media infrastructure controlled by the tech sector.
The ensuing analysis isolates different emotions circulated by AI hype, including doomsday hype, drawing on examples from the 2020s AI hype cycle. The article concludes by examining the ethics of promotional culture as part of the combined knowledge apparatus supporting value construction in AI.
AI hype, promotional culture, and affective capitalism (Clea Bourne, University of London, May 2024)
In rushing from A (Alpha) to E (Equity/Exit), they skipped the crucial steps of B (Building safe systems), C (Careful evaluation), and D (Demonstrating alignment), while fundamentally misunderstanding what it means to develop a new technological "alphabet" that might reshape human society.
Does the rush to deploy generative AI represent a collective failure to grasp the profound social implications of rewriting how humans communicate with information and each other?
An industry building systems that take shortcuts to optimise reward functions. Will this wave of generative AI model makers fall victim to the same mechanisms?