This is building on a range of papers that look at overeliance on GenAI. Two of which I explored on 14th February in context of widespread dependence on retail vs enterprise models delivered via apps or the web vs APIs.
That may not be immediately apparent, but please bear with me, I'll get there.
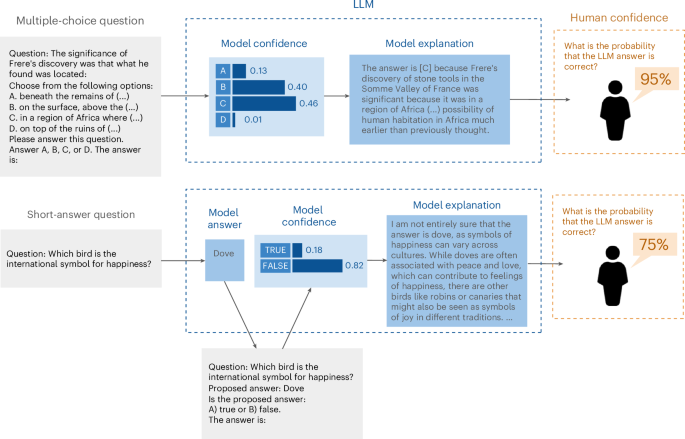
When we rely on anything for something that needs to be accurate, or something with an associated impact, we need to validate output. We need people who have learned to recognise what good looks like in any given usage context.
Because I said so, or because the AI said so
Custer's last stand for people at the end of their knowledge, time, or patience tether. A lifelong right of passage. Sometimes there are building blocks that can't be placed until later.
Don't cross the road on red is a fair 'Because I said so' moment. Especially if a small person is in kamikaze mode. Debates on road safety and situational exceptions have to wait when there is imminent risk of child purée. They can wait until little plastic brains have more means to balance motivations and implications.
Growing up and watching others grow there will always be some homework paralysis. Periods of acute doubt. Periods of grinding to a halt. Not able to pierce the fog. Not wanting to waste time down inaccurate cul-de-sacs.
If part A of equation B is wrong, then solution C will be off. If explanations of personification were opaque, the essay could be building on mistakes. If French lessons were murky, speaking tests might be a stammering mess.
Copying others' homework can be really tempting. A lot hinges on the information and people to hand.
The usual parental advice, when available, is that mistakes are one of the most important parts of learning. If a child gets everything right (maybe due to great pattern matching or photographic recall), there is little muscle memory built to navigate when limits of that capability are reached.
Being wrong, processing that, reversing, diagnosing, finding good people to explain things, recognising good sources. Those are life skills.
No-one can know everything. People make sense of things in different ways. Poor teachers don't flex to find the right mental hooks for individual students. Great teachers often don't have time for that. Teaching has to homogenise to maximise throughput. Sometimes that is the commercially motivated objective.
Those who persistently question and seek more clarification (the 'Why? and 'How?'' kids) are at risk of being viewed as aberrations. 'The rest of the class gets it, so why don't you?'
But do they?
Those that quest to really 'get' stuff. Those who can't rest easy with 'Because I said so' or 'Because the AI said so'. Those kids are our scarce resources, but they need a network of trusted sources. People to help them reverse engineer, find foundations, create 'Aha!' moments, then build back.
I'm willing to bet it's those moments that get most teachers out of bed.
Does GenAI offer that?
Whether with basic things (How do I make brownies?), more involved things (How do I code this UX component?), truly complex things (Does this variable contribute causally to climate change?), or immediately impactful things (Should this person receive benefits?), there are some fundamental parts of the hypothesis, research, analysis, thesis, peer-review, translate for practical action process missing.
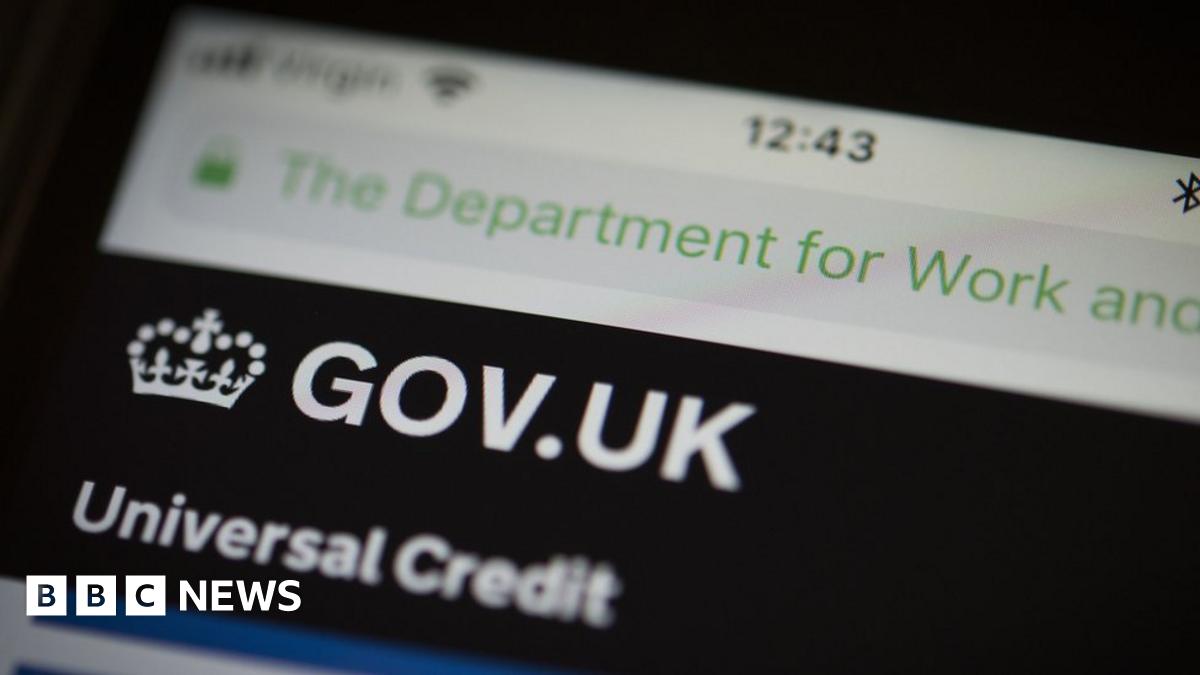
This is a release in beta, fail fast, regroup and iterate technology cycle with potentially huge negative impacts that are yet to be well understood.
Is it useful?
It depends
Sometimes that is perfectly fine. Mostly when fallout is minimal, extremely rare, or easily reversible.
We hear consistent reports of GenAI doing things. Of course we do, it is a general purpose technology in search of the killer monetisable applications.
What we hear less about, excepting anecdotal examples, is when it goes wrong, and what was tried to fix it.
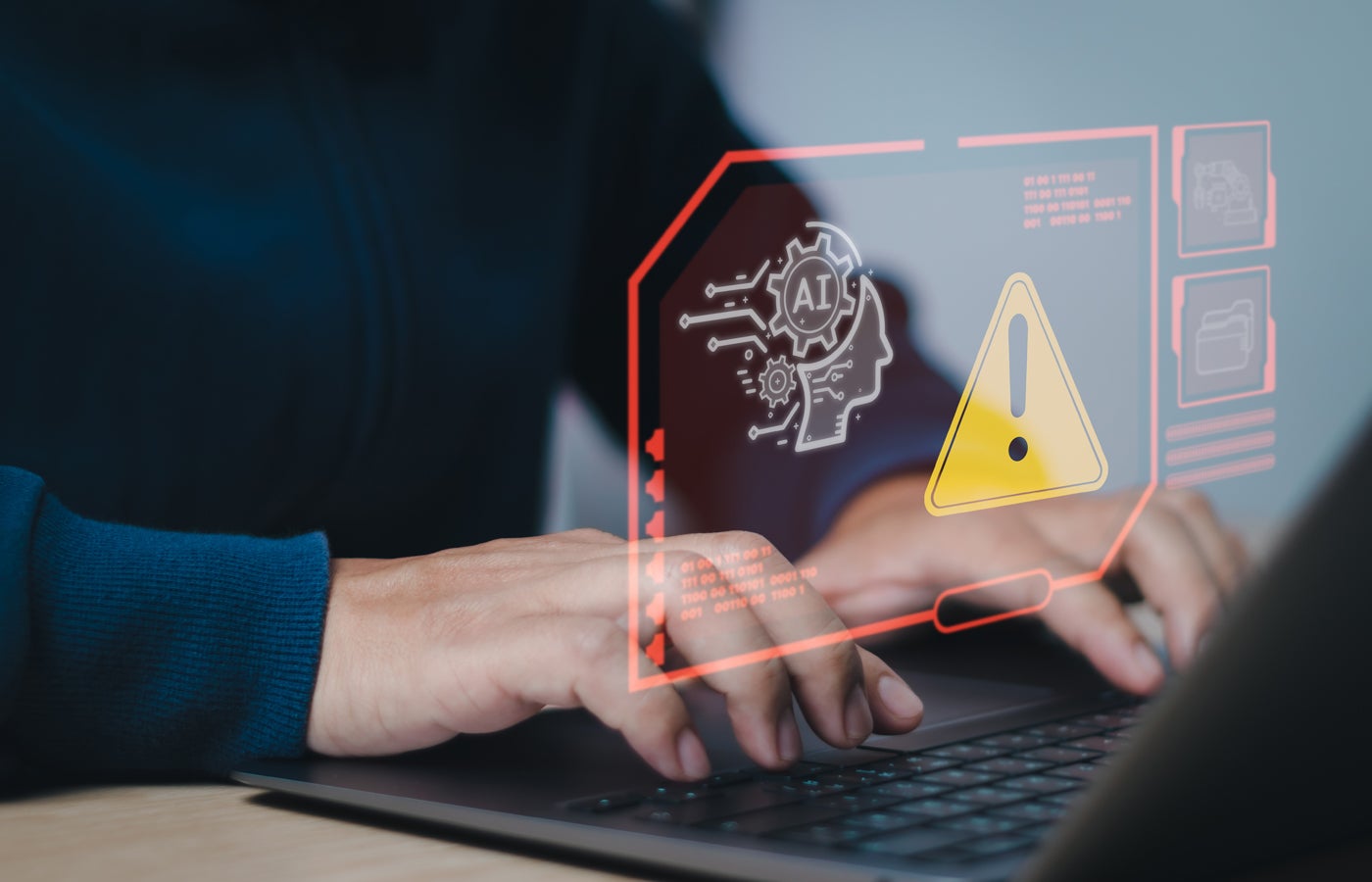
What we rarely hear about are the times implementation is rolled back or abandoned. Of course, as the evangelising goes, you used it wrong, you didn't try hard enough, or that particular version of the tool, with those specific components, and that specific data, with that kind of prompting, on your platform, sitting on that infrastructure, was not fit for that purpose.
What we hear least about is alternatives. Where tweaks to pre-existing systems, more warm bodies, better quality data, revised processes, or less novel tools do the job better.
Back to those continuous learners
Our kid in 'Why?' and 'How?' mode is falling behind. All of their peers are using GenAI to help with homework.
Their teachers stress the need to use it as a supportive tool, not as an oracle. They prohibited cutting and pasting. They deployed increasingly pointless AI detection tools, built out from old plagiarism detection engines.
All while teachers use loss-leader AI plug ins to various platforms and retail tools to design lesson plans, do some marking, and experiment with AI supported student evaluations.
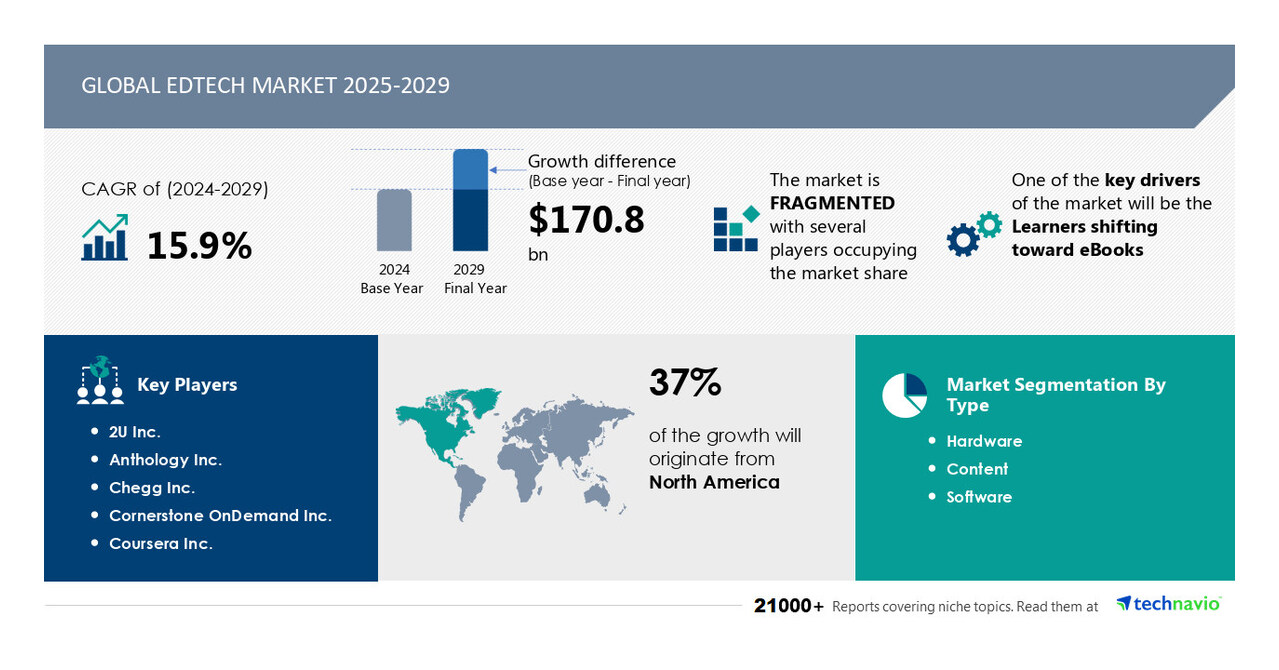
They are also, under government mandate, teaching classes about AI potential. AI / ML basics, prompting techniques, creative uses. Ways it might alter the whole world of work, without ever quite drilling to the nuts and bolts of 'Why?' And 'How?'.
They struggle to address instances of inappropriate AI memes and AI porn. Not really clear about the scale of such content on social media platforms.
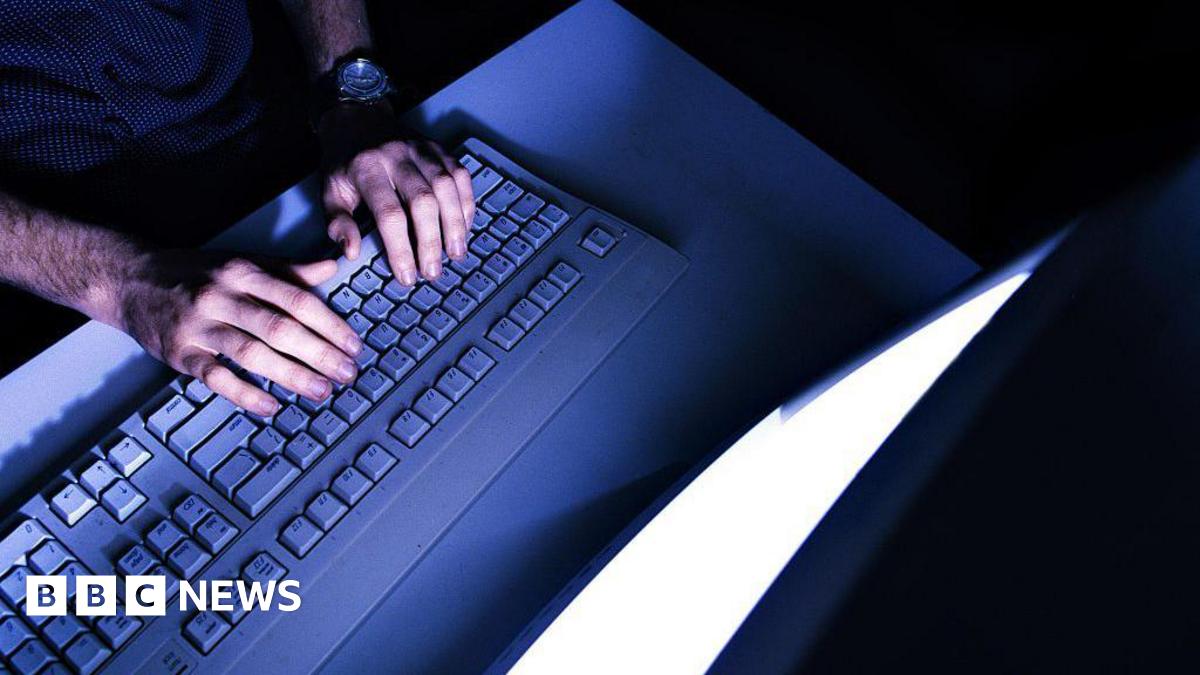
Showing is better than telling, so an entire lesson plan on the Holocaust is put together using AI. The kids are told it is ChatGPT and Copilot output. They have to trust the teacher checked all sources. Lots about it looks 'off'.
They get sent off to do the group work. Their own presentation, using GenAI, on one aspect of the Holocaust.
The words and slides are not great. Their internet native familiarity with tools is helping, but the teacher is just a beginner. Heck, everyone is a beginner, this has only been available at any scale for just over two years.
The kids give in and redo the slides without AI. When asked why one said:
"It's the Holocaust"
The old beyond their years subtext was a duty felt to get it right, not to find shortcuts. They focused on work to check facts vs tweaking AI output.
The subject matter and implications, the value in working through and understanding sources, was just too important to make do and hit the 'good enough' button.
Other groups, not so much. They hit the brief. They hit send. Some errors were subjectively hilarious, if you didn't scratch the historical surface.
Why was it wrong Miss?
The teacher had no really useful answer for this beyond some hand waving about training data and the probabilistic nature of most outputs.
How can we fix it? That's a full stop and vendor manuals don't get into that. Not for specific usage contexts.
That's down to your due diligence and choices. Down to your means, motive, and opportunity to scrupulously assess tool capability, accuracy, security, data protection, and ethical implications of contextual usage.
A long read from UNESCO on balancing those considerations.
AI first US government systems
We have a lot of things that will happen in the next wee while. AI from the world's richest man is reportedly being inserted into government systems that control an unimaginable array of things that can affect billions.
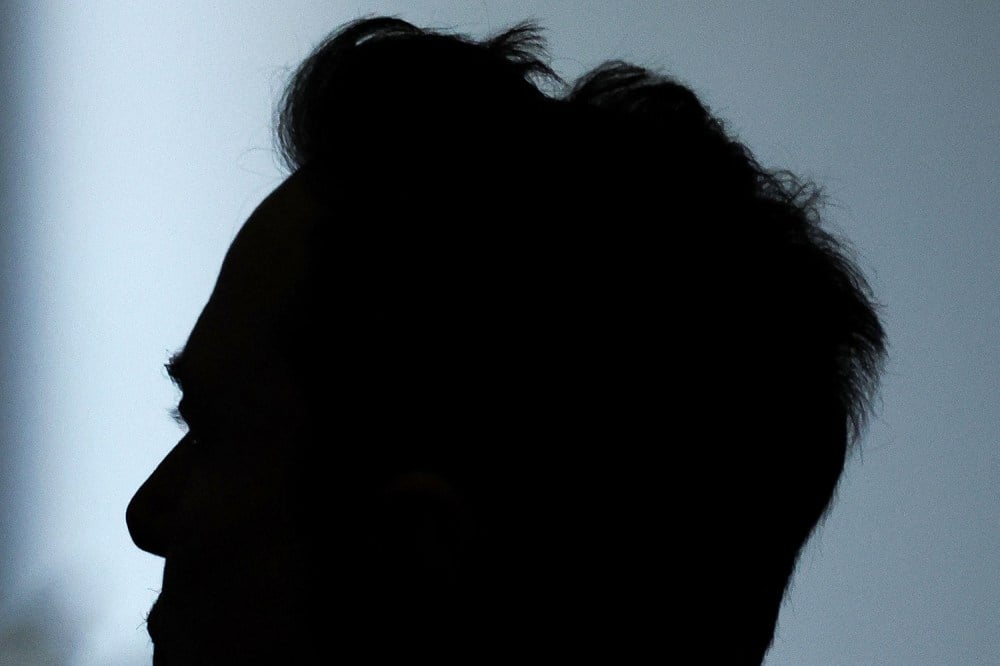
Firing of probationers, some of whom are the most capable staff who just changed role or got promoted and so entered another probationary period.
There have been blanket emails asking for voluntary resignations. Keyword searches for signs of being D, E, I, A, climate change response, or otherwise 'woke' policy advocates as filtering mechanism, allegedly using AI to sort people, content, and spend destined for removal.
All being done with little to no consideration of implications, but the advertised aim is wiping out government debt, excising the deep state, and returning power to the people. While the recent Republican budget increases the debt by $4-ish Trillion and reportedly delivers over 80% of the tax cuts to the top 0.1% of the population.
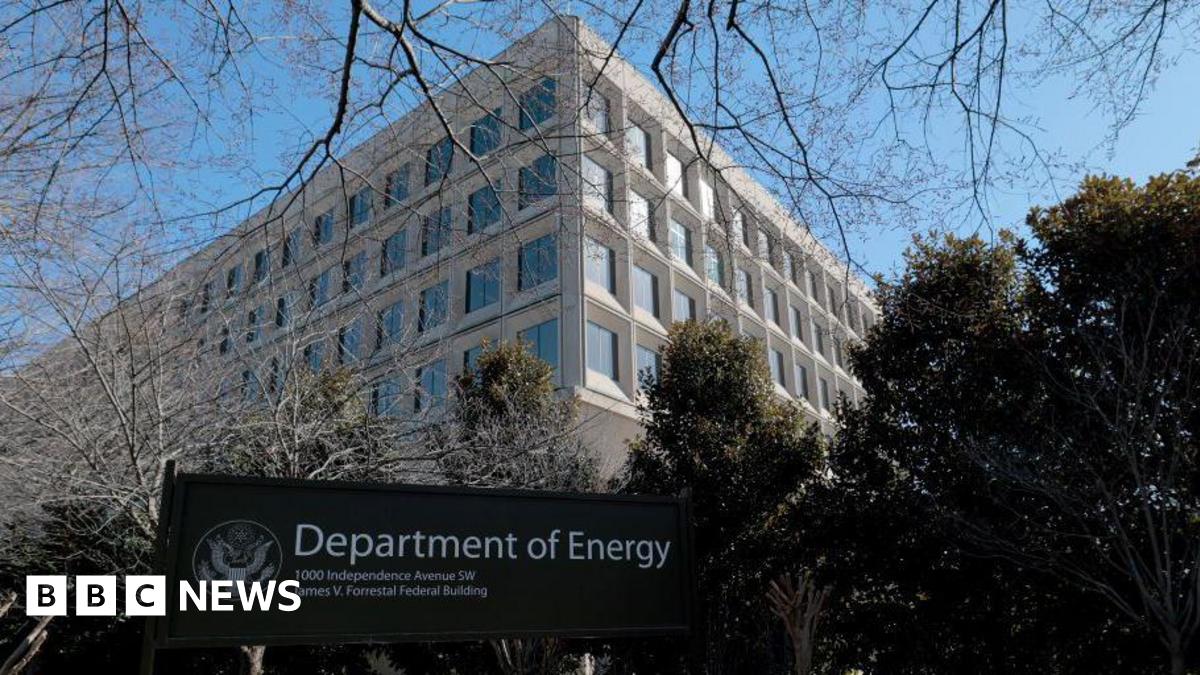
This will make everything better
We need our 'How?' and 'Why?' kids more than ever. Toddler like tenacity to question aims, methods, outcomes, and workings out in this equation.
The kids who reject tech solutionism, looking at the full context.
Will GenAI help to do that? We will see, but I doubt it. The next generation is being subjected to the biggest global FAFO experience, while being taught that AI will save us.
But it's the Holocaust
There is a lot to learn from that statement. Not, as it happens, a made up story. There are plenty of global factions who will say it never happened, both the story about the kid, and the Holocaust.
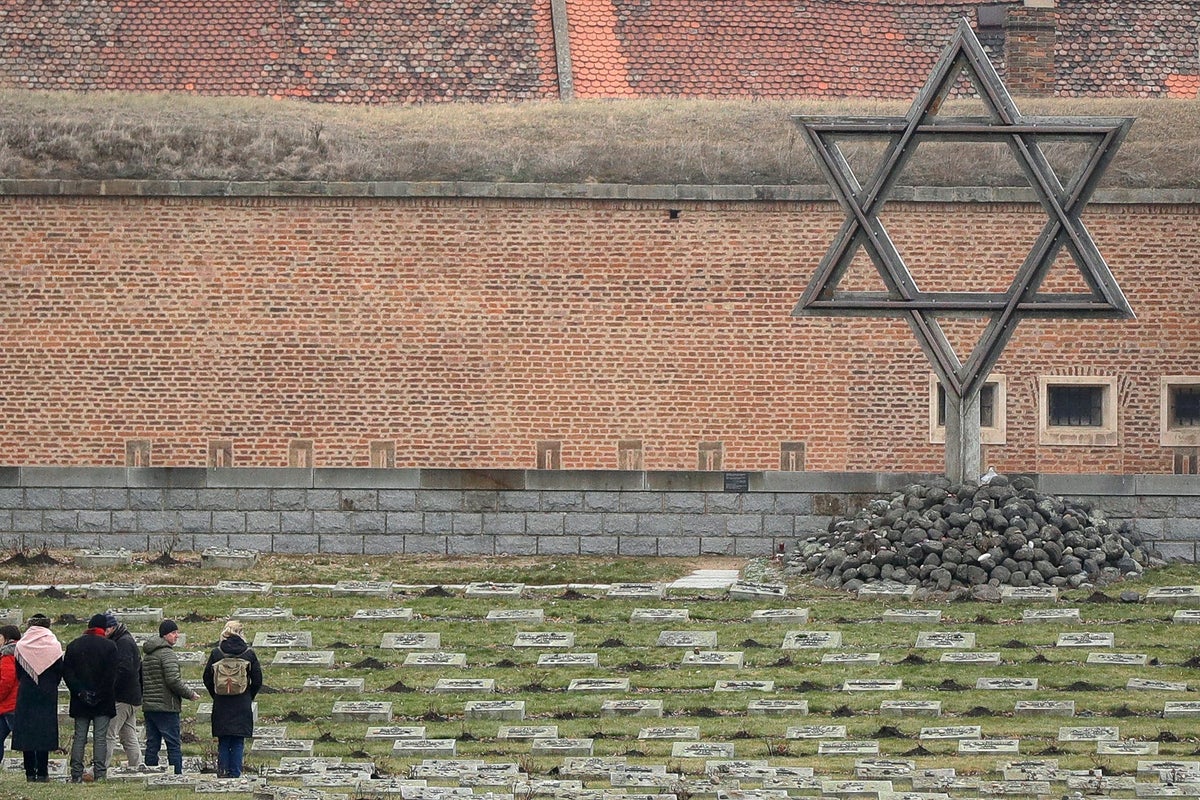
First person accounts of history (or best secondary sources) are going to have to be closely guarded. What will the next generation of AI train on, either through proprietary choice or data availability? Grok-3 looks interesting - if you ignore the organisational and founder ethos. Following on from DeepSeek R1, what are the trade offs people tolerate for more speed, bells, whistles, and / or lower token bills?
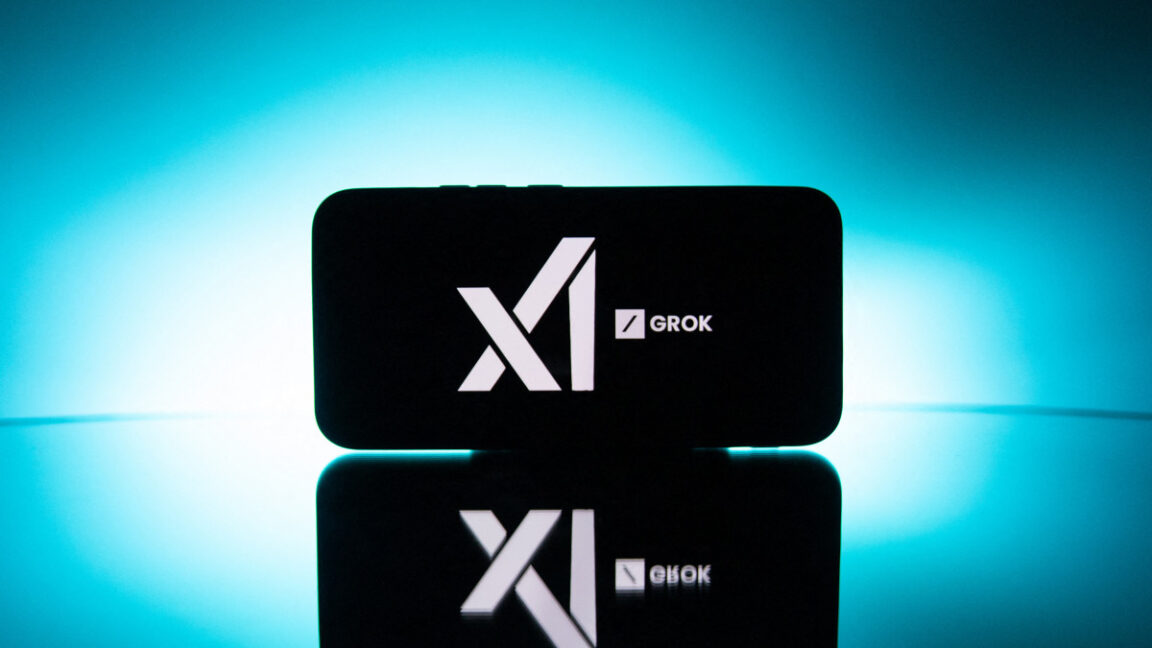
All good questions that we need to support our children to ask. While we fight for access to accurate information to be able to give a good answer. Resisting, on their behalf, effort to corrupt and remove sources.
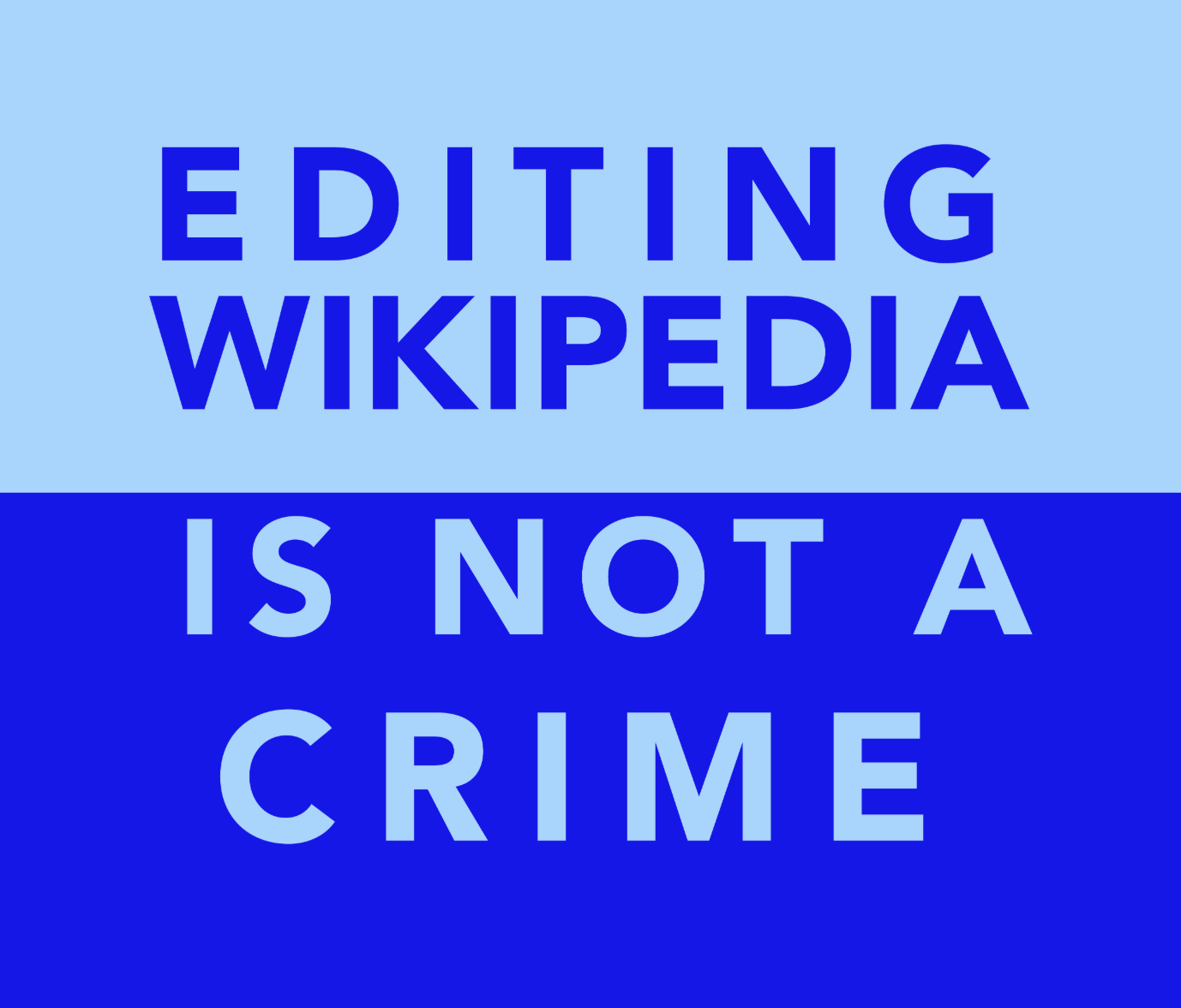
Then ask me again about the nett value equation for this wave of AI.