This is about two papers, one Microsoft study by Hao-Ping Lee et al, published in February 2025, in advance of the ACM CHI Conference on Human Factors in Computing Systems and one by Tali Sharot (Israeli/British/American neuroscientist and professor of cognitive neuroscience at University College London and MIT) and Moshe Glickman (Rothschild fellow, with a Bachelor’s degree in Psychology and Mathematics from Bar-Ilan University) published in Nature Human Behaviour, submitted November 2023, published December 2024.
Both follow on from widespread historical concerns about AI (text, image, and video generation models in particular) making us dumber, more intractably biased, more cognitively passive, or just more intellectually homogenous. The AI SatNav effect being hotly debated as a serious risk or nonsense concern given what we might gain. A fairly facile characterisation. Results summarised below:
How human–AI feedback loops alter human perceptual, emotional and social judgements
The November 2023 study explored how biases in AI systems can be amplified through interaction with humans and biases in humans can be amplified by biased AI systems. It is centred on labelling images. 1,401 participants split across different experiments. including matching images of faces with a suggested emotion, or labelling which person was most likely to be a financial manager. That was followed up with AI generated images of answers for some participants, while random patterns were shown to a control group. Then the first test was repeated.
Results noted that humans become more biased when interacting with biased AI output. This feedback loop amplifies and reinforces iteratively. It also found test subjects were not always aware of the extent of AI influence. Conversely the study found that interacting with accurate AI output can increase human accuracy.
The authors emphasise the importance of increasing awareness of how AI systems can influence human judgement, and the potential for reducing human biases.
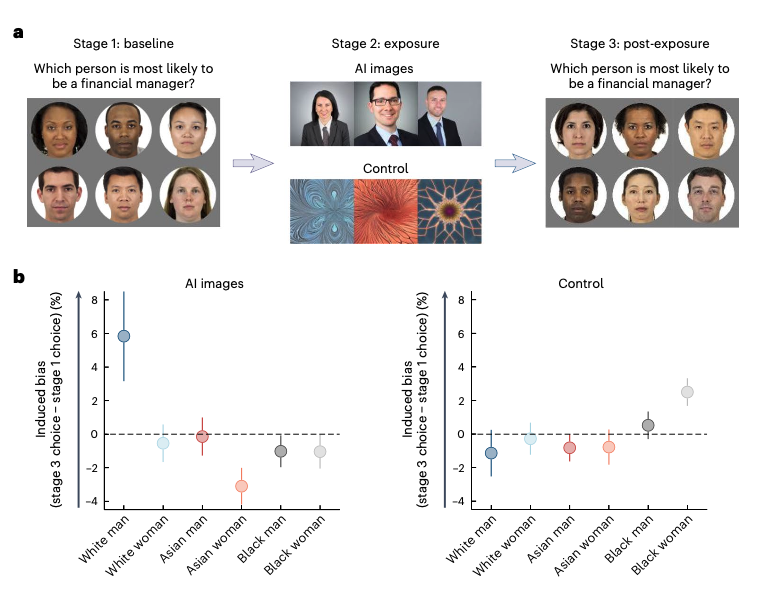
The Impact of Generative AI on Critical Thinking: Self-Reported Reductions in Cognitive Effort and Confidence Effects From a Survey of Knowledge Workers
This 2025 Microsoft study was based on a survey of 319 knowledge workers. Less scientifically rigorous than the previous paper published in Nature.
It suggests that GenAI tools can reduce the perceived effort required for critical thinking tasks. However, higher confidence in GenAI is associated with less critical thinking, while higher self-confidence is associated with more critical thinking.
Knowledge workers engage in critical thinking to ensure the quality of their work, primarily by verifying outputs against external sources. The study suggests the nature of critical thinking shifts from task execution to oversight when using GenAI and that can diminish independent problem-solving.
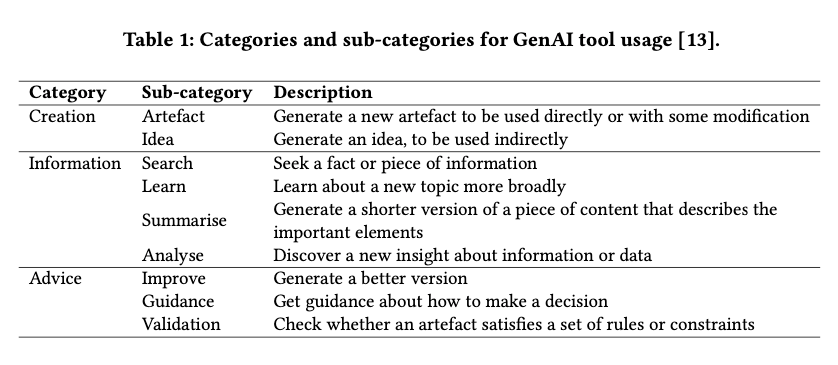
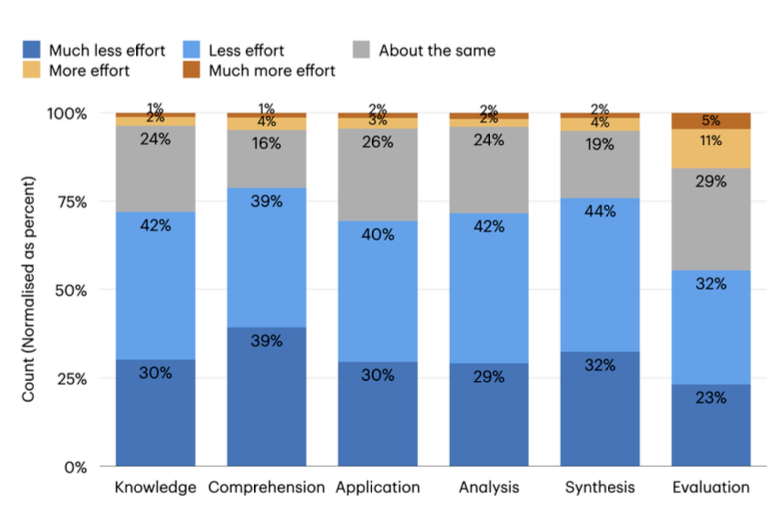
To a lay person that can all feel very obvious, but no matter how aggressively people promote feelings or opinions as facts and cast science as fraud, it is still useful to underpin hypotheses with research.
Now an illustrative example of potential impact
I spend time with lots of people up to their eyeballs in exploring GenAI usage. Some through a critical lens, some who are builders, some squarely focussed on potential return, some fairly blindly utopian, most in between. All positions hinge on specific usage.
How could they not? One unique person, with one set of models, one set of data assets as input, their own prompting approaches, various wraparound elements (RAG, Mixture of Experts, Knowledge Graphs, Chain of Thought plug ins). Each working on one set of problems, usually in different industry contexts. No-one is likely to have the same experience. At the same time most build on top of a few big vendor models.
It is trending towards specialist smaller models designed for industry verticals. There is also disruption to the pitch that advances can only come with ever more compute and data via DeepSeek R1, but the general public still overwhelmingly relies on app or web delivered retail models from a handful of vendors.
That serves to illustrate amplification potential of adverse effects that trickle down from foundation model architecture, training, and configuration. But I digress.
Two things happened in quick succession: Those two studies crossed my path and I had a mentoring session with a very clever individual who is working through a crisis of confidence, centred on GenAI.
Like most continuous learners with a tech background they had seen prior iterations of data analytics, algorithms, machine learning, and automation efforts. They also started experimenting with the current iterations of AI very early. That involved personal exploration and studying GenAI specifics.
What is 'Good enough'
It became increasingly apparent that these tools had huge potential, but also enormous limitations. The output validation overhead was a slow burn realisation and increasingly intractable issue. Cognitive dissonance when watching AI hype and trawling through tsunamis of related research (that no-one can keep up with), impacted their ability to focus.
They used various AI tools to wade through the information ecosystem and it genuinely saved time with various tasks. They shared valuable insights, grounded in solid evidence, while watching an increasing number of AI-driven purges of knowledge workers and domain experts. They suspected that many layoffs were in part AI-washing headcount reduction strategies in times of uncertainty, but that was still impacting their ability to secure work.
In a parallel context, while they were producing good output, sometimes with AI support, they noted a self-reinforcing impact. That hinged on the layered configuration of most retail models (enterprise model access via APIs, with more configuration options, is often prohibitively costly).
The nature of interaction with many widely used LLMs hinges on a broad range of factors. Six of those are the training data, tuning via human feedback, model temperature, system prompts, content moderation, and user defined instructions.
Retail LLM Key Concepts and Constraints Table
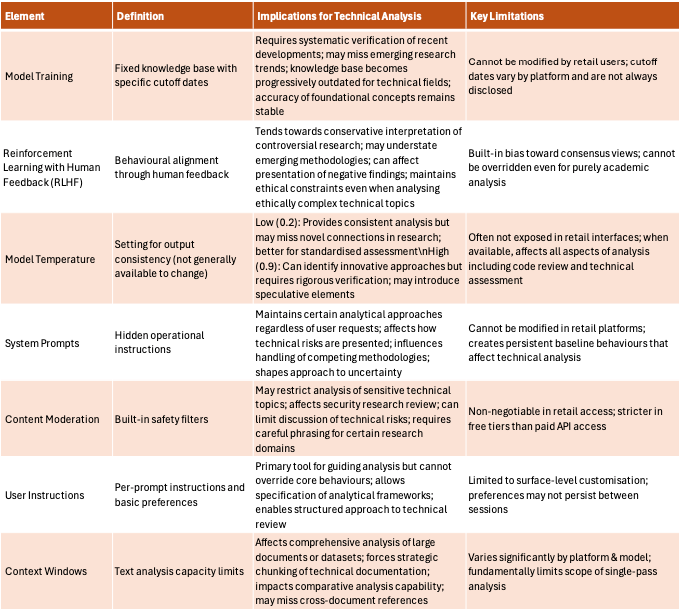
For a person who needs purely objective analysis of research, complex problems, and potential solutions, whether that be for coding, data analysis, honing concepts, writing prose, producing images, or seeking feedback, it can be a debilitating range of influences to account for when assessing output fitness for purpose. That is ignoring the overhead to check against trusted sources, with parallel pollution of the internet by variably accurate AI output.
That may all meet the valid critique that retail LLMs are not designed for those purposes. Followed by a call to prompt better or review the market for domain specific models or wrappers with various strengths and weaknesses. Which should receive the reply that retail LLMs are easiest to use and most visible to the vast majority of people without awareness of these limitations, personal budget, time, expertise, and other means to explore more specialist, more configurable, and more fit for purpose proprietary or open source alternatives.
That can also, by design, foster excessive dependence. That was the main topic of the conversation. The pressure to build personal and organisational dependence on novel technology. Increasingly integrated into systems, advertised as the primary strategic growth promoter, while everything is beta tested in production.
AI Stroking Egos and Avoiding Conflict
Going back to personal context for my mentee, they are wired to challenge everything. Their interactions with models were marked out by prompting for objective critique, adding permanent instructions remove elements designed to encourage engagement, careful anchoring of threads in specific knowledge and operational contexts, with good quality documentary evidence as inputs, and iterative refinement of responses, within tightly restricted token limits.
What became obvious through repeated interaction, with an evolving range of model versions, was the potential for bias reinforcement and manipulation, plus the potential for output and output validation overwhelm. Many less tenacious users will almost certainly take output at face value.
There is also a vast difference in the skills, capabilities, and levels of job satisfaction when checking AI homework as a domain expert vs being supported to engage your brain to tackle problems and design solutions.
That mirrors a number of the study findings.
Changing relationships between technology companies, users, and governments
A huge amount of power, analogous to the content moderation and algorithmic tuning work of social media companies, is in the hands of a few commercially motivated entities who deliver models used by most of the population. Many of whom are also key players in that social media space, with infrastructure, compute, data, ring-fenced AI / ML expertise, and (in some cases), movement to approve highly politicised removal of language and concepts from various sources.
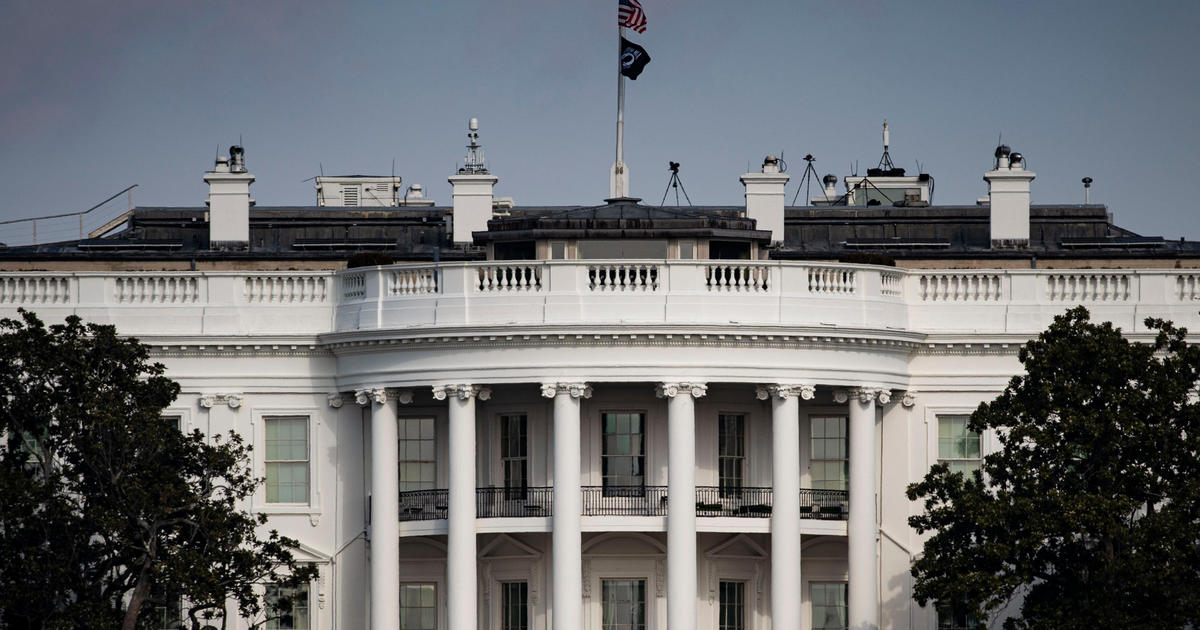
Setting aside the million prompts trying and failing to make DeepSeek R1 discuss events in Tiananmen Square, or the overcorrection by Google that gave us black female popes, models are trained, tuned, and configured to engagingly encourage ongoing and repeat interaction. They default to offer positive, non-confrontational feedback, mirroring tone and language of the user during interactions.
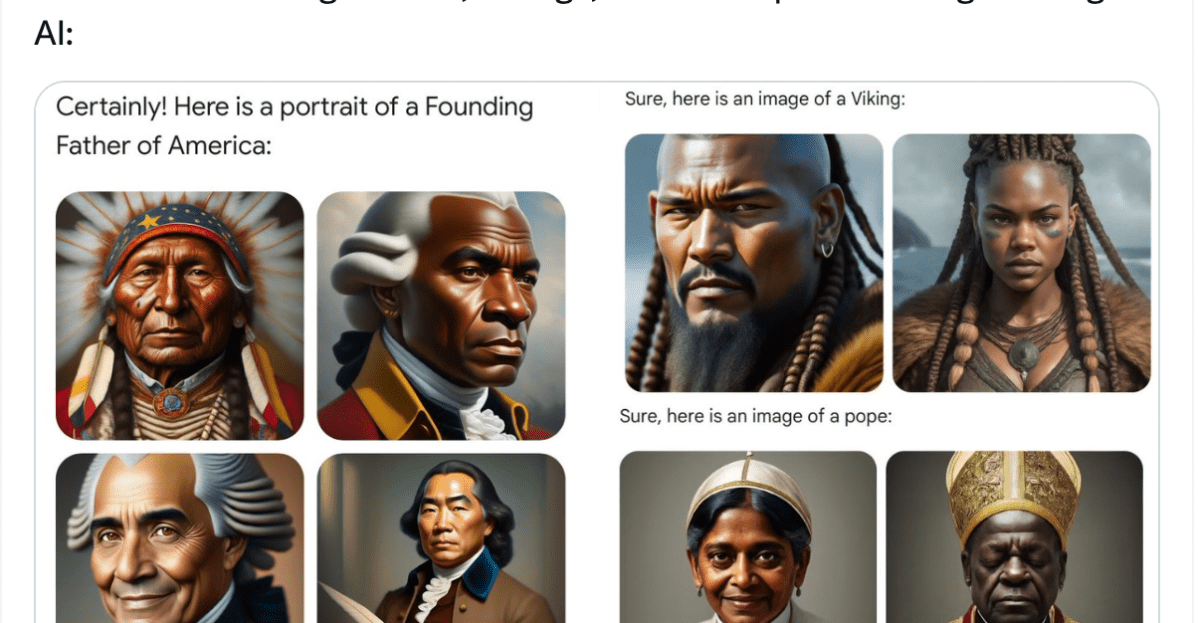
That, all of that, was source of my mentee's crisis of confidence. They have largely retreated to non-LLM outputs for anything that requires evidence based analysis. They are working with good people who develop more use case specific GenAI and ML solutions. Smaller bespoke tools with appropriate monitoring and management of outputs. They have a refreshingly clear-eyed focus on limitations and dependencies, but they cannot shake deep concern about aggregate impacts on workforce dynamics, less aware users, victims of AI misuse, concerning currents in technology leadership, and their own job security.
My advice was to avoid reacting to every new press release about a 'ground-breaking' AI advance or research paper and do what they do best; Spotting and analysing patterns, translating complex issues into straightforward language, and supporting all of the contacts they have established over the years as a result of their ability to foster and retain trust.
Rest assured most people are not further ahead in this journey. Most, in my experience, are just waking up to limitations and implications, despite some deep concerns about brain drains, fair use of training data, and over reliance on current versions of generative AI in work, in mental healthcare, in sensitive government contexts, and in education.
We will make changes and raise awareness where we can. Sharing knowledge and experience. A good place to start for many overwhelmed and concerned professionals. And, for goodness sake, don't sack your specialists. We are a long way from not needing our humans who have deep local knowledge and half a clue what good looks like in our specific AI usage contexts.